
This work represents the data-driven ML models using a Bayesian network (BN). Therefore, improving ML models’ interpretability is essential to maximize the acceptance of such approaches in these emerging, fast-growing, and promising application fields. The black-box nature of most ML models hinders their applications in important decision-making domains like autonomous vehicles, the financial stock market, or e-health. In IoT applications, the transparency of the model is essential for better interpretability and reliability. The Internet of Things (IoT) field is expanding faster than its supporting technologies. However, unclear reasoning mechanisms behind an automatic decision often represent one of ML approaches’ drawbacks: this feature is typically referred to as interpretability or explainability of the model. Nowadays, ML is extensively used for making accurate predictions in several application areas such as medicine, stock markets, criminal justice systems, telecommunication networks, and many others. Machine learning (ML) theories and applications experienced tremendous growth in the past few decades. The capability of a model to allow evaluation of abnormalities at different levels of abstraction in the learned models is addressed as a key aspect for interpretability. Each vehicle is considered an example of an Internet of Things (IoT) node, therefore providing results that can be generalized to an IoT framework where agents have different sensors, actuators, and tasks to be accomplished. As a case study, abnormality detection is analyzed as a primary feature of the collective awareness (CA) of a network of vehicles performing cooperative behaviors.
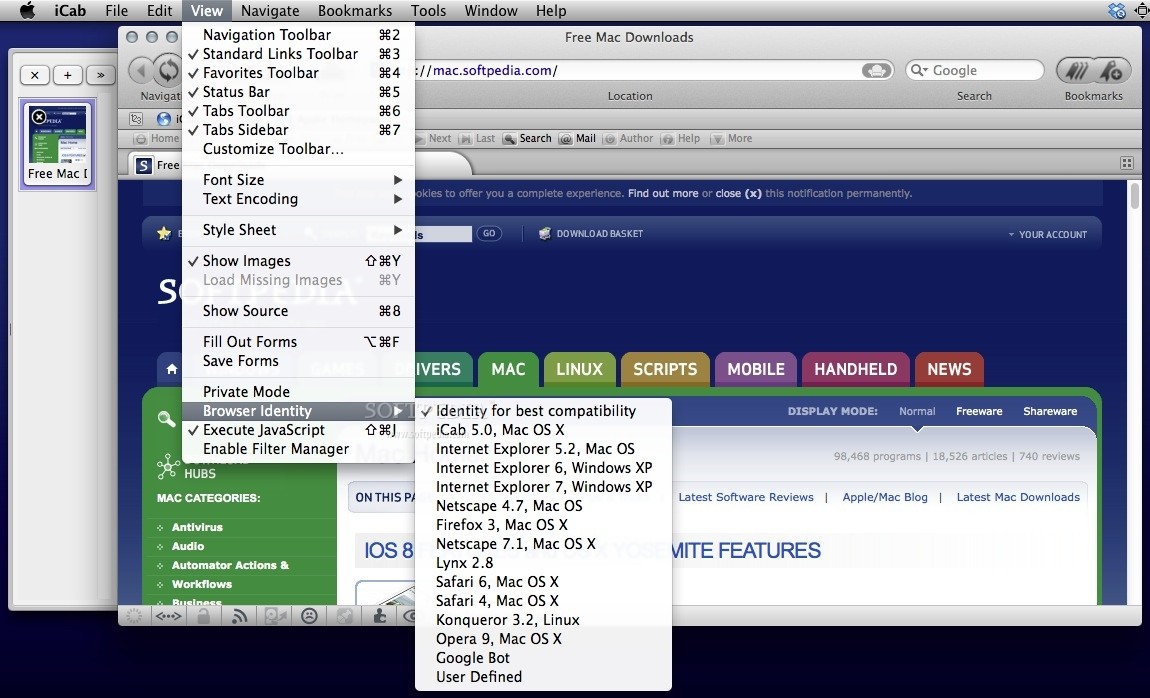
It is demonstrated that the capability of incrementally updating learned representation models based on progressive experiences of the agent is shown to be strictly related to interpretability capability. The proposed approach assumes that the data-driven models to be chosen should support emergent self-awareness (SA) of the agents at multiple abstraction levels. The interpretability in this work is achieved through graph matching of semantic level vocabulary generated from the data and their relationships. This paper concentrates on one of the core functionality of such systems, i.e., abnormality detection, and on choosing a model representation modality based on a data-driven machine learning (ML) technique such that the outcomes become interpretable. The necessity of interpretability is often related to the evaluation of performances in complex systems and the acceptance of agents’ automatization processes where critical high-risk decisions have to be taken. The interpretability of ML models can be defined as the capability to understand the reasons that contributed to generating a given outcome in a complex autonomous or semi-autonomous system.
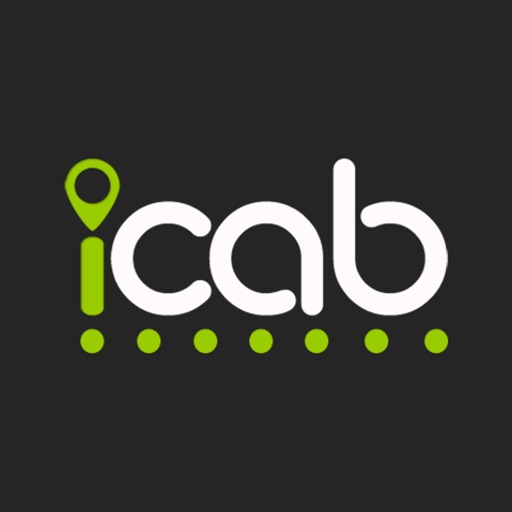
In recent days, it is becoming essential to ensure that the outcomes of signal processing methods based on machine learning (ML) data-driven models can provide interpretable predictions.
